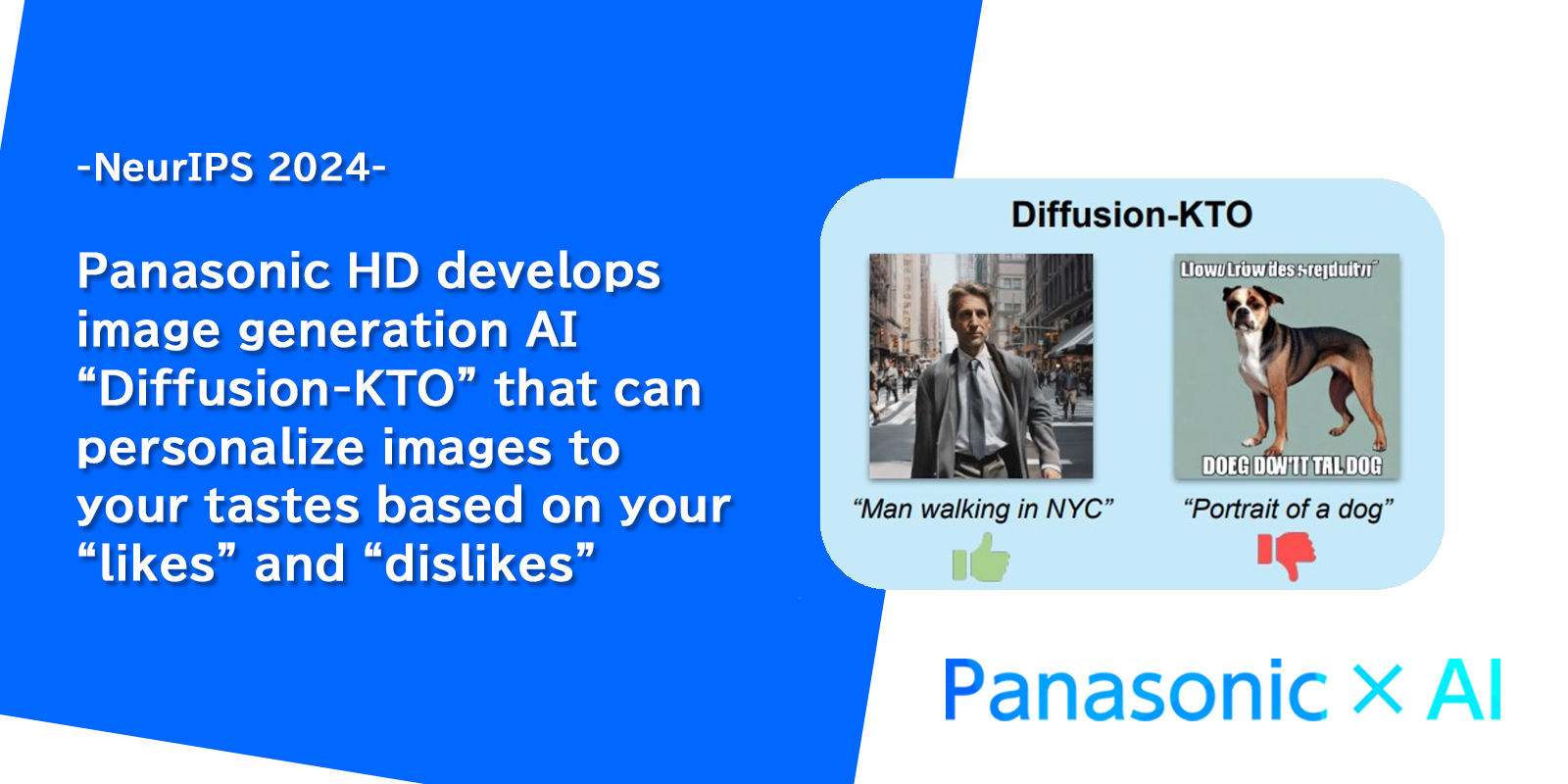
Dec 02, 2024
- Company
- Press Release
- R&D
- North America
- AI & Robotics
Nov 21, 2023
Company / Press Release
Osaka, Japan – Panasonic R&D Company of America, in collaboration with UC Berkeley, has leveraged prior knowledge of large-scale language models to build a new multimodal foundation model “HIPIE” that can perform image recognition and segmentation in response to any text input. “HIPIE” achieved the State-of-the-art performance*1 in evaluation experiments for over 40 public datasets.
In recent years, many research fields have attempted to introduce large-scale language models, and they are attracting attention as they can reduce the time and cost required to build AI models.
When incorporating the prior knowledge of a large-scale language model into an image, if hierarchical languages of different granularity (e.g., person -> face -> nose/mouth/eyes, etc.) exist in the same object region, basically, hierarchical relationships have been ignored, and domains and languages have been treated as having a one-to-one correspondence (one of the following: person/face/nose, or simply connecting “person, face, nose”) learning with strings, etc.).
We have focused on the fact that “hierarchical relationships”, which have been ignored, are essential for advanced image understanding. We have developed a new architecture that learns hierarchical representations of different granularity, making it possible to perform segmentation and image recognition with a single model, which previously required multiple models depending on the hierarchy. This will be expected to reduce the burden of on-site annotation in a variety of situations where hierarchical image understanding is required, such as danger detection with in-vehicle sensors, recognition of operating targets in robots, topographical analysis by drones, and medical images.
This technology has been internationally recognized for its advancedness and has been accepted by the 37th Conference on Neural Information Processing Systems (NeurIPS 2023), the top conference for AI and machine learning research (acceptance rate 26.1%). This research will be presented at the plenary conference to be held in New Orleans, USA from December 10, 2023 to December 16, 2023.
*1: As of November 21, 2023, according to Panasonic data.
As AI technology advances, there is a growing need for technology that enables more advanced image understanding in a wide range of fields such as autonomous driving, robotics, and factories. Among these, image segmentation has been actively researched and developed as an important task in the computer vision field due to its wide range of applications. However, pixel-by-pixel annotation is time-consuming and costly, which is an issue in social implementation.
In recent years, with the rapid development of natural language processing technology, many methods have been proposed in the field of computer vision that incorporate prior knowledge of large-scale language models and perform fine-tuning using small amounts of additional training data for each desired task. Research and development in this field has progressed rapidly over the past few years, as it has become clear that accurate AI can be achieved without preparing the large amount of training data that was previously required.
However, incorporating large-scale language models into computer vision and successfully handling images and text remains a challenge. For example, consider an example of a person in an image in segmentation or image recognition task. The person area would normally be assigned the “person” label. Also, depending on the task, it may be necessary to assign labels with different granularity to the region, such as “face” or “nose”.
When learning labels with different granularity at the same time, it is common to train the label names by connecting them, such as “person nose” and “dog nose”. However, AI trained using this method had the problem that it could not handle unknown text such as “giraffe nose”.FF If the representation of the model is not rich as in this case, it cannot be said that there is a deep understanding of the image.
The newly developed HIPIE (Hierarchical Open-vocabulary Universal Image Segmentation) focuses on this issue and is a technology that learns labels by hierarchy and can comprehensively handle labels with different granularity. By encompassing various scales, it enables desired image segmentation and advanced image understanding, as shown in Figure 1. The model's representation has greatly improved, making it possible to deal with unknown combinations of labels as mentioned earlier.
Figure 1 Output results of HIPIE, which performs hierarchical image segmentation according to image and text input (quoted from the accepted paper)
Figure 2 shows the HIPIE configuration. First, image features (MAE) and text features (BERT) are extracted from the input image and text, and their correspondence is learned using Bi-Directional Cross-Attention, a method that can learn the degree of bidirectional association. (Text-Image Fusion).
Here, the object region is a relatively small continuous region surrounded by boundaries, while the background region tends to be widely distributed in an irregular shape throughout the image. In HIPIE, image features and text It is possible to quantitatively compare the characteristics of features and appropriately divide the object region and background region. Furthermore, by processing the object region and background region using different decoders, we were able to achieve more effective image understanding.
Figure 2 HIPIE configuration diagram (quoted from the accepted paper)
Figure 3 shows a table comparing the tasks that can be handled by HIPIE and typical segmentation models such as SAM, SEEM, and ODICE. HIPIE is a technology that can perform the eight types of segmentation and object detection tasks described in one model. Additionally, in evaluation experiments using over 40 public datasets, our method achieved better results than conventional methods.
Figure 3 Comparison of corresponding tasks between this method (HIPIE) and representative segmentation models such as SAM, EEM, and ODICE (quoted from the accepted paper)
HIPIE is a new multimodal foundation model with the following characteristics: open vocabulary (can be used in any language), universal (can be used with any image), and hierarchical (information contained in a scene can be captured hierarchically) .
It enables us to understand scenes reflected in images in detail, and it is expected to be used in situations that require advanced image understanding, such as mobility, manufacturing, and robotics. It is also expected to become a technology that lowers the hurdles for building and training basic models, which are in increasing demand these days.
Panasonic will continue to accelerate the social implementation of AI technology and promote research and development of AI technology that will help customers in their daily lives and work.
Hierarchical Open-vocabulary Universal Image Segmentation
https://arxiv.org/abs/2307.00764
This research is led by Konstantinos Kallidromitis of Panasonic R&D Company of America and the University of California, Berkeley as equally contributing first authors, with Yusuke Kato and Kazuki Kozuka of Panasonic Holdings Technology Division as co-authors.
About the Panasonic Group Founded in 1918, and today a global leader in developing innovative technologies and solutions for wide-ranging applications in the consumer electronics, housing, automotive, industry, communications, and energy sectors worldwide, the Panasonic Group switched to an operating company system on April 1, 2022 with Panasonic Holdings Corporation serving as a holding company and eight companies positioned under its umbrella. The Group reported consolidated net sales of 8,378.9 billion yen for the year ended March 31, 2023. To learn more about the Panasonic Group, please visit: https://holdings.panasonic/global/ |
The content in this website is accurate at the time of publication but may be subject to change without notice.
Please note therefore that these documents may not always contain the most up-to-date information.
Please note that German, French and Chinese versions are machine translations, so the quality and accuracy may vary.