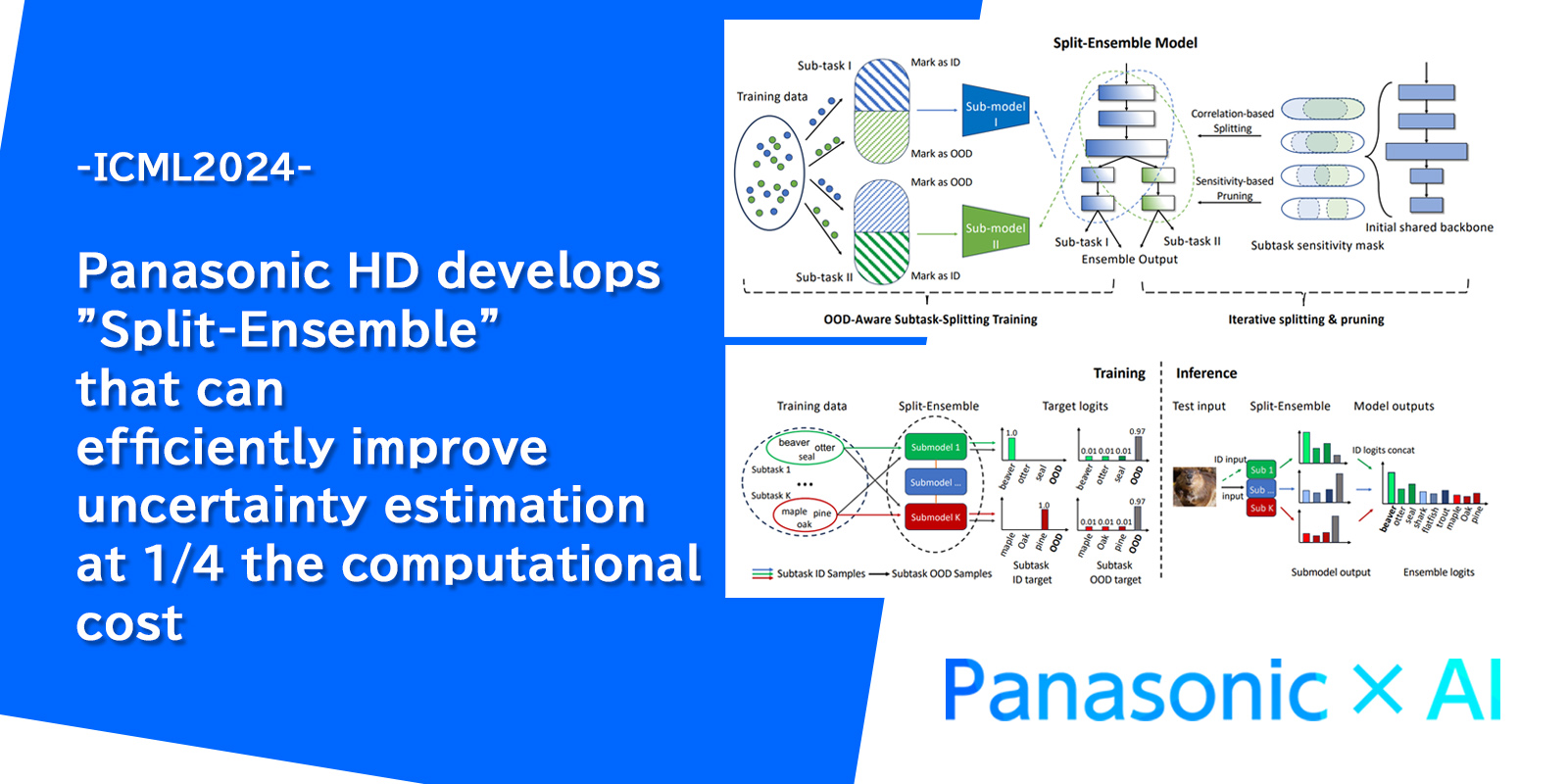
Jul 17, 2024
- Company
- Press Release
- R&D
- AI & Robotics
Sep 22, 2023
Company / Blog Posts
Osaka, Japan – Panasonic Holdings Corporation is the first in the field of computer vision to clarify the relationship between variational inference and self-supervised learning*1, and to develop an image recognition AI that understands image features while estimating image uncertainty.
AI “understands” the attributes of objects in images by learning from large datasets.
A challenge in developing highly accurate models is the need to manually label large datasets. But in recent years, advances have been made in developing ways for AI to learn on its own from large amounts of unlabeled data, known as self-supervised learning (SSL). SSL has led to major advances in the field of natural language processing, as seen in the recent overwhelming progress of GPT. On the other hand, large-scale datasets often contain highly uncertain data that is difficult to judge even when viewed by humans (due to things such as noise, blurring, or light reflection), which prevents AI from learning. This uncertainty reduces the quality of AI, so it has attracted a lot of attention in recent years as a problem that needs to be solved.
Our method is the first to theoretically integrate variational inference and self-supervised learning to achieve AI that can predict not only images’ features, but also their uncertainty, which has been difficult in the past.
In addition, through experiments, we have demonstrated that it is possible to estimate the uncertainty of features in images (the degree to which an image is difficult for AI to learn), which has been quite difficult in the field of self-supervised learning. This technology is expected to be used in a wide range of fields in the future to solve the issues of data quantity and quality required for AI learning and to increase the reliability of AI.
This technology has established a theory that provides an overview of self-supervised learning algorithms, and has been internationally recognized for its academic contribution and advancedness, having also been accepted in International Conference on Computer Vision (ICCV) 2023 (with an acceptance rate of 26.15%), one of the top conferences in the field of computer vision research. The presentation will be made at the plenary conference in Paris, France from October 2 to October 6, 2023.
*1 As of September 22, 2023
Developing image-based AI models capable of tasks such as recognition, detection, and segmentation requires a significant amount of time and cost to collect large amounts of data and prepare training data through annotations. This is a major issue in the social implementation of AI.
Therefore, in recent years, self-supervised learning has been actively developed as a method to significantly reduce the annotation load. Self-supervised learning uses pseudo labels generated by the AI itself in advance from a large amount of unlabeled data to learn image features, then achieves the desired task with high precision using a small amount of data for each task. SimSiam, SimCLR, and DINO are well-known conventional methods.
When pre-training features that appear in images from a large amount of unlabeled data, to obtain a general feature representation that can be applied to a variety of tasks, AI needs to learn so that it can recognize a given object even if the object appears in various different forms, such as when the image is cropped or rotated, or is lighted differently.
With self-supervised learning such as the aforementioned SimSiam, image augmentation such as rotation, cropping, and color conversion are automatically performed on each image, and the distances between image features of these augmented images are calculated automatically. In the pre-training phase, AI is trained by minimizing the distance of image features of the given object, which enables AI to recognize the object as the same given object even if it looks different. It is known that with SSL can be used for various tasks with high precision with a small amount of labeling.
However, conventional self-supervised learning does not take into account the properties of each image when learning.
Images with high uncertainty and images with low uncertainty are treated the same way, which may cause issues in the pre-training phase or accuracy of the model. Panasonic HD attempted to solve this problem using a probabilistic statistical approach. Probabilistic generative models such as Variational Auto Encoder are known to be good in expressing uncertainty. In this paper, we demonstrated that the formulas used in conventional self-supervised learning can be derived from the formulas of this Variational Auto Encoder, and theoretically clarified the relationship between them (Figure 1).
Furthermore, we developed a method that can estimate the uncertainty of each image in datasets. In an evaluation experiment on ImageNet100 (benchmark dataset), we qualitatively demonstrated that our method was able to estimate the uncertainty of images (Figure 2), and we obtained quantitative findings that, in classification tasks, images estimated by this method to have high uncertainty tend to have a low percentage of correct answers, which indicates uncertainty affects the recognition rate of AI (Figure 3).
Until now, it has been common knowledge that a large amount of high-quality data is required for AI training data, but our research showed that the quality of training data may be treated as an uncertainty. We were able to demonstrate the possibility of realizing AI that can overcome the hurdle of data quality by incorporating estimated certainty into the AI algorithm.
Figure 1 Relationship between this method (VI-SimSiam), conventional self-supervised learning methods such as SimSiam, SimCLR, and DINO, and the formulas of stochastic generative models. It is shown that different algorithms can be derived by changing the definitions of probability distributions p and q in a stochastic generative model. (Quoted from the accepted paper © 2023 IEEE)
Figure 2 Uncertainty of images estimated using this method. The upper row were estimated to be images with low uncertainty, and the lower row were estimated to be images with high uncertainty. (Quoted from the accepted paper © 2023 IEEE)
Figure 3 Histogram showing the relationship between the parameter κ indicating uncertainty and image recognition accuracy. As κ increases and uncertainty decreases, the percentage of correct answers increases, and the image recognition accuracy shown by the solid line also increases. (Quoted from the accepted paper © 2023 IEEE)
Our self-supervised learning algorithm can learn not only image features, but also their uncertainties without the need for laborious human labeling of large data sets, so it not only solves the problem of data volume, but also may enable AI to handle data quality, an essential for AI development.
Panasonic HD will continue to accelerate the social implementation of AI technology and promote research and development of AI technology that will help customers in their daily lives as well as at work.
Representation Uncertainty in Self-Supervised Learning as Variational Inference
https://arxiv.org/abs/2203.11437
This research is the result of a collaboration with Hiroki Nakamura and Masashi Okada of the Panasonic HD Technology Division, and Professor Tadahiro Taniguchi of Ritsumeikan University/ Panasonic HD Technology Division.
ICCV2023
https://iccv2023.thecvf.com/
Panasonic×AI WEB
https://tech-ai.panasonic.com/en/
Founded in 1918, and today a global leader in developing innovative technologies and solutions for wide-ranging applications in the consumer electronics, housing, automotive, industry, communications, and energy sectors worldwide, the Panasonic Group switched to an operating company system on April 1, 2022 with Panasonic Holdings Corporation serving as a holding company and eight companies positioned under its umbrella. The Group reported consolidated net sales of 8,378.9 billion yen for the year ended March 31, 2023. To learn more about the Panasonic Group, please visit: https://holdings.panasonic/global/
The content in this website is accurate at the time of publication but may be subject to change without notice.
Please note therefore that these documents may not always contain the most up-to-date information.
Please note that German, French and Chinese versions are machine translations, so the quality and accuracy may vary.