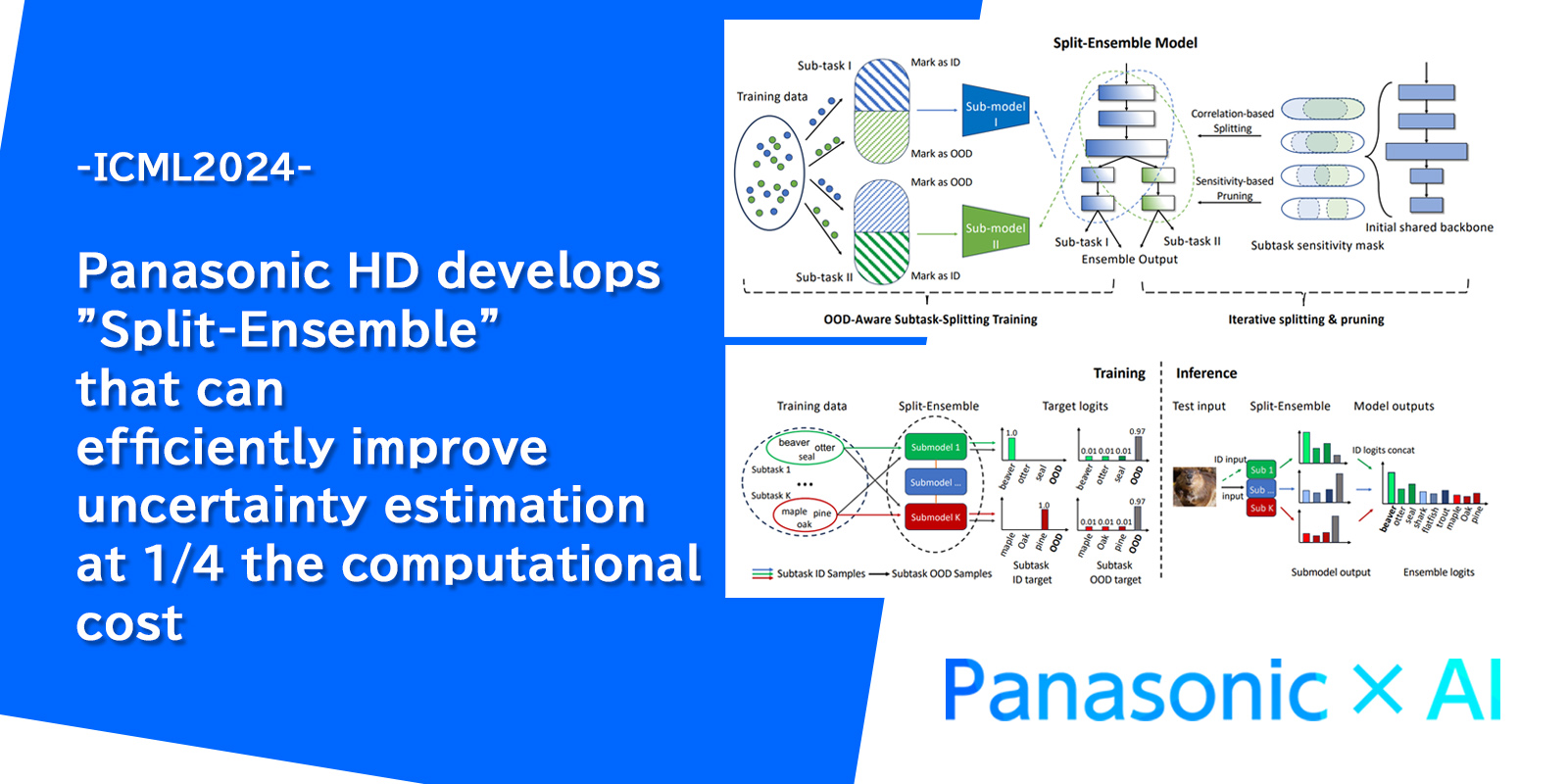
Jul 17, 2024
- Company
- Press Release
- R&D
- AI & Robotics
Feb 16, 2024
Company / Press Release
The technology uses one-third the number of parameters compared to previous methods
Osaka, Japan – Panasonic Holdings Co., Ltd. (Panasonic HD) has jointly developed an Adverse weather removal AI with researchers from the University of California, Berkeley (UC Berkeley), Nanjing University, and Peking University that improves image recognition accuracy by removing rain, snow, fog, and other elements from images that can significantly reduce image recognition accuracy. This method demonstrated image restoration performance that improved recognition accuracy over conventional methods while saving over 72% of parameters and 39% of inference time in image recognition and segmentation tasks for multiple adverse weather images.
Outdoor image recognition AI applications are becoming increasingly common in fields such as mobility and infrastructure. However, rain, snow and fog can drastically alter the appearance of objects in images captured outdoors, significantly reducing image recognition accuracy. In recent years, the task of erasing rain, snow fog and other elements from images, known as “weather removal,” has gained steam as a means to enable practical AI for use in all weather conditions. Until now, methods have been proposed to prepare different models depending on the type of weather, or to integrate models so that they can be used in all weather conditions, but the large number of calculations required had proven a bottleneck for these methods.
By representing different weather parameters as weights, the joint development team created a technology that can reliably remove the influence of adverse weather using a small number of parameters in a single model that can handle multiple weather elements and tasks. This proposed method could be used in a variety of situations where high-precision image recognition is required in all weather conditions, such as danger detection systems for in-vehicle sensors and security cameras.
The technology has been internationally recognized and accepted into the 38th Annual AAAI Conference on Artificial Intelligence (AAAI 2024), a top conference for AI and machine learning technology, and will be presented at the conference, to be held in Vancouver, Canada from February 22, 2024 to February 25, 2024.
Figure 1 Schematic diagram of the adverse weather removal AI.
With the advancement of AI technology, there are an increasing number of situations in which robust image recognition is required even outdoors, such as in the mobility and infrastructure fields. However, outdoor environments present many variables that cannot be controlled. In particular, rain, snow and fog can drastically alter the appearance of objects in images captured outdoors, significantly reducing image recognition accuracy, which is an issue in industrial applications. Research and development of adverse weather removal tasks such as raindrop removal and fog removal (“dehazing”), is actively underway.
Efforts to build AI models (“expert models”) specialized for specific weather conditions and tasks are showing some results. However, in the real world, multiple adverse weather elements may be present at the same time, and methods capable of more reliable judgment are being sought. Therefore, research has been conducted on ensemble models that mix multiple expert models, but since the number of parameters increases dramatically, no practical model exists in terms of computational complexity.
In order to solve the above issues, the joint development team created “Mixture-of-Feature-Modulation-Experts” (MoFME). This weather removal AI can remove rain, snow, and fog, which degrade image recognition accuracy, with a single ensemble model and with one-third the number of parameters compared to conventional models.
Figure 2 the results of adverse weather removal and segmentation using MoFME for the dataset “RainCityscapes”
Two new components have been introduced in this AI to accomplish tasks such as image recognition and segmentation, which previously required multiple expert models depending on the weather and task, with a single ensemble model and with a practical amount of calculation.
The first is the “Feature Modulated Expert,” which expresses the parameters of multiple expert models using linear transformation weights. Rather than learning the parameters of different expert models individually, the AI represents them through linear modulation of a specific expert model, reducing the total number of parameters and the amount of calculation.
The second is an “uncertainty-aware router” that switches the contribution of each expert model according to the characteristics of the input image. Each expert model specializes in different weather tasks. If an expert model is not very confident in its weather removal results, it can be told to reduce its contribution, and vice versa. This effectively increases the reliability of the ensemble model and improves image recognition performance.
Figure 2 shows the results of adverse weather removal and segmentation using MoFME for the dataset “RainCityscapes.” The MoFME method removed both rain and fog, even for a complex image that contained both of these elements, and obtained results equivalent to a clear image. In addition, in the segmentation after removing adverse weather, the adverse weather-removed image preprocessed using the MoFME method achieved the best results in terms of segmentation.
The MoFME method demonstrated image restoration performance that improved recognition accuracy over conventional methods while saving over 72% of parameters and 39% of inference time in image recognition and segmentation tasks for multiple adverse weather images.
MoFME reduces the impact of rain, snow, fog, and other adverse weather elements, which can significantly reduce image recognition accuracy, on image recognition AI performance by removing these elements from images. Since MoFME can cleanly remove rain and fog with one-third the number of parameters compared to conventional methods, it can be used in situations that require robust image recognition even outdoors, such as in the mobility and infrastructure fields.
Panasonic HD will continue to accelerate the social implementation of AI technology and promote research and development of AI technology that will help customers in their daily lives and work.
Efficient Deweather Mixture-of-Experts with Uncertainty-aware Feature-wise Linear Modulation
https://arxiv.org/abs/2312.16610
This technology was developed under the framework of the “BAIR Open Research Commons*” led by UC Berkeley and is the result of research in which Denis Gudovskiy of Panasonic R&D Center America and Tomoyuki Okuno and Yohei Nakata of Panasonic HD Technology Division participated.
* An AI research institute established as a place for the world's top researchers to openly collaborate across the boundaries of industry and academia. As of February, 2024, 10 companies are participating, including Panasonic HD, Google, and Meta.
- AAAI2024
https://aaai.org/aaai-conference/
- Panasonic×AI website
https://tech-ai.panasonic.com/en/
About the Panasonic Group Founded in 1918, and today a global leader in developing innovative technologies and solutions for wide-ranging applications in the consumer electronics, housing, automotive, industry, communications, and energy sectors worldwide, the Panasonic Group switched to an operating company system on April 1, 2022 with Panasonic Holdings Corporation serving as a holding company and eight companies positioned under its umbrella. The Group reported consolidated net sales of 8,378.9 billion yen for the year ended March 31, 2023. To learn more about the Panasonic Group, please visit: https://holdings.panasonic/global/ |
The content in this website is accurate at the time of publication but may be subject to change without notice.
Please note therefore that these documents may not always contain the most up-to-date information.
Please note that German, French and Chinese versions are machine translations, so the quality and accuracy may vary.