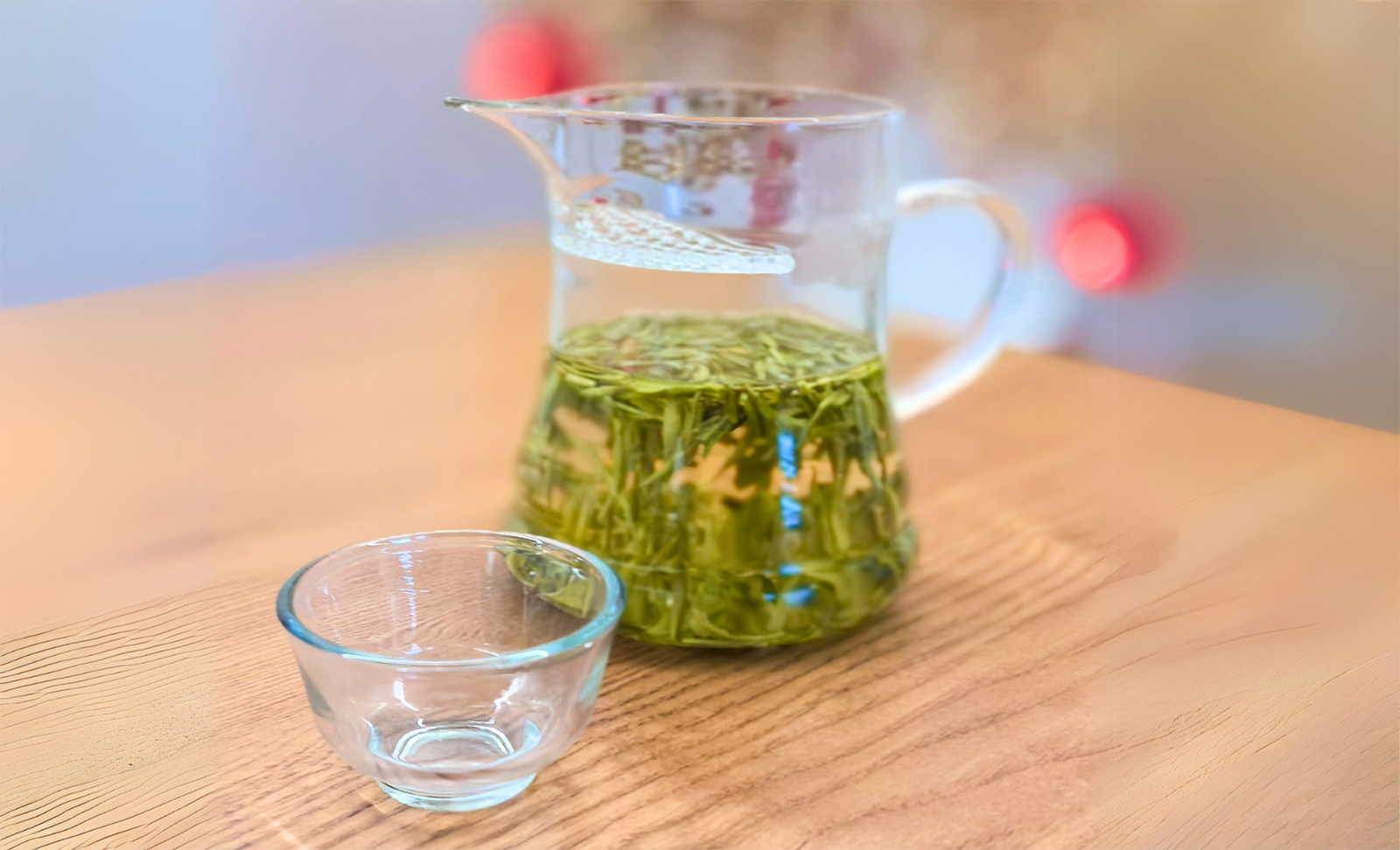
Apr 03, 2025
- Company
- Press Release
- R&D
Jul 17, 2024
Company / Press Release
Osaka, Japan - Panasonic Holdings (Panasonic HD) has developed a “Split-Ensemble” method which can efficiently improve uncertainty estimation and out-of-distribution (OOD) detection performance, together with researchers from the University of California, Berkeley (UC Berkeley), Peking University, and Carnegie Mellon University. Experiments showed the effectiveness of Split-Ensemble compared to conventional methods.
The reliability of AI is becoming increasingly important. In particular, the problem of an AI being unable to make a judgment on its “unknown” data space could lead to fatal risks depending on the use case. To address this issue, we developed the “Split-Ensemble” that divides a task to be solved by an AI into multiple subtasks that classify similar objects and creates the “unknown data” from multiple perspectives within the complementary subtasks, thereby efficiently acquiring the ability to distinguish unknown data.
“Split-Ensemble” has been accepted at the 41st International Conference on Machine Learning (ICML 2024), a top conference in the fields of AI and machine learning (acceptance rate 27.5%), and will be presented at the conference, which will be held in Vienna, Austria from July 21 to July 27, 2024.
Recently, image recognition and detection AI are supporting human decision-making in a wide range of fields. On the other hand, the inability of AI to properly handle unknown data and situations, i.e., out-of-distribution (OOD) data that AI has not learned, leads to the risk of misjudgment, and there are many use cases where the impact can be fatal, for example, in autonomous driving. Also, recently, hallucinations*1 caused by a lack of OOD detection capabilities have become a problem even in large language models (LLMs), and appropriate measures to address OOD are needed.
When dealing with OOD, conventional methods required preparing external data to be trained as OOD and incurring additional computational costs. In contrast, Split-Ensemble allows you to deal with OOD using multiple sub-models created only from existing datasets. Specifically, we prepare multiple sub-tasks for distinguishing similar objects, and train multiple sub-models to solve each task (Figure 1). In the sub-tasks, the architecture is such that some data is treated as in-distribution data (ID), and the rest is trained as out-of-distribution data (OOD). For example, by setting categories such as “beavers, otters, sea lions, and everything else (OOD)” and “maples, oaks, pines, and everything else (OOD),” the AI can learn how to distinguish between similar categories as well as how to distinguish between OOD categories (Figure 2).
Figure 1: Schematic diagram of Split-Ensemble (quoted from the accepted paper)
Figure 2: Diagram explaining division into subtasks (quoted from the accepted paper)
To solve the original task, it is necessary to train sub-models on multiple sub-tasks and ensemble their outputs, but the amount of calculation required increases as the number of models to be trained increases, even for small-scale models. Therefore, in this study, we have suppressed the increase in the amount of calculation by using techniques such as sharing backbones between sub-models and pruning models.
When we verified the effectiveness of this method using benchmark datasets (CIFAR-10, CIFAR100), the result showed that Split-Ensemble can distinguish OOD with a quarter of the computational cost compared to conventional methods*2. In addition to its high performance, the fact that there is no need to prepare and train separate OOD data is a major advantage in the development process.
Split-Ensemble is a method that can deal with OOD with only a quarter of the computational cost of conventional methods, and is expected to be useful in various situations where OOD challenge needs to be addressed. The greatest impact of this method is that its unique architecture improves the OOD detection ability of deep learning models. In this work, we evaluated it on relatively small-scale tasks such as the ImageNet dataset, but we believe that applying this method to large-scale LLMs will lead to the suppression of hallucinations in the future.
Panasonic HD will continue to accelerate the social implementation of AI and promote research and development of AI technology that will help customers in their daily lives and work.
“Split-Ensemble: Efficient OOD-aware Ensemble via Task and Model Splitting”
https://arxiv.org/abs/2312.09148
This technology was developed within the framework of the BAIR Open Research Commons*3 led by UC Berkeley, and is the result of research participated by Denis Gudovskiy of Panasonic R&D Center America, and Tomoyuki Okuno and Yohei Nakata of Panasonic HD Technology Headquarters.
Additionally, within the above-mentioned BAIR Open Research Commons framework, the following paper, collaborative research between Panasonic HD and researchers from UC Berkeley, ITALAI S.R.L, and Sapienza University of Rome, was also accepted for ICML 2024. Targeting semantic segmentation tasks under domain shifts, the paper proposes an active learning method that exceeds the performance of supervised domain adaptation with just 1% of the labels, accelerating the deployment of AI in the field.
“Hyperbolic Active Learning for Semantic Segmentation under Domain Shift”
https://arxiv.org/abs/2306.11180
Also, the following paper, collaborative research between Panasonic HD and researchers from UC Berkeley, Nanjing University, and Peking University, was accepted for the ICML'24 Workshop on Advancing Neural Network Training (WANT): Computational Efficiency, Scalability, and Resource Optimization, a workshop held alongside ICML 2024. The paper proposes a new method for prioritizing the prevention of accuracy degradation in particularly important categories (people, cars, etc.), which are referred to in the paper as “task-critical,” when reducing model weight through model quantization.
“Fisher-aware Quantization for DETR Detectors with Critical-category Objectives”
https://arxiv.org/abs/2407.03442
Note:
*1: The problem of AI boldly giving answers that cannot be justified by the data it has learned
*2: Naïve Ensemble
*3: An AI research institute established as a place for the world's top researchers to openly collaborate across the boundaries of industry and academia. As of July, 2024, 10 companies are participating, including Panasonic HD, Google, and Meta.
About the Panasonic Group Founded in 1918, and today a global leader in developing innovative technologies and solutions for wide-ranging applications in the consumer electronics, housing, automotive, industry, communications, and energy sectors worldwide, the Panasonic Group switched to an operating company system on April 1, 2022 with Panasonic Holdings Corporation serving as a holding company and eight companies positioned under its umbrella. The Group reported consolidated net sales of 8,496.4 billion yen for the year ended March 31, 2024. To learn more about the Panasonic Group, please visit: https://holdings.panasonic/global/ |
The content in this website is accurate at the time of publication but may be subject to change without notice.
Please note therefore that these documents may not always contain the most up-to-date information.
Please note that German, French and Chinese versions are machine translations, so the quality and accuracy may vary.