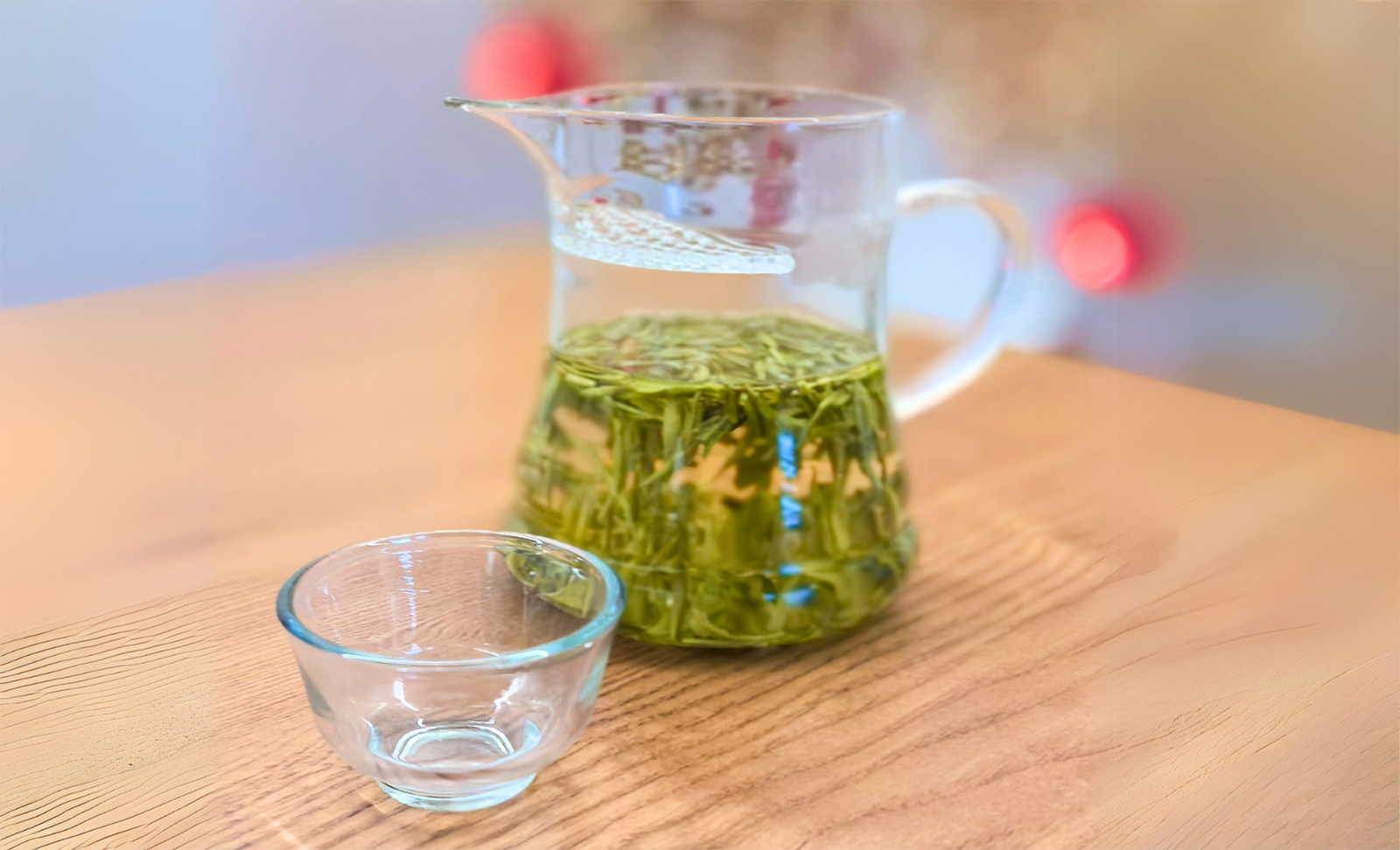
Apr 03, 2025
- Company
- Press Release
- R&D
Jul 28, 2023
Company / Press Release
Efforts towards Responsible AI
Osaka, Japan - Panasonic R&D Company of America and Panasonic Holdings Corporation have developed a technology that increases the reliability of the AI model by detecting objects that the AI model has not learned and that are essentially unrecognizable as "unknown objects."
The image recognition AI model accurately recognizes objects that are trained in advance, but it is difficult to train the image recognition AI model with all objects in the real world. Therefore, in the actual usage environment, it is inevitable that the AI model will face unknown objects.
On the other hand, AI models are not good at judging what they have not seen as "unknown." In recent years, with the progress of social implementation of AI, it has become a major issue that AI models forcibly recognize and "pretends to know" what they have never seen, and treat it as something AI models know. This can lead to unexpected malfunctions.
While technology to increase the reliability of AI is attracting attention, we have developed a new flow-based generative model “FlowEneDet” that enhances reliability simply by extending a pretrained segmentation model with it. Proposed flow-based detector with an energy-based inputs enables a low-complex architecture that correctly recognizes only objects that have already been trained and are inherently recognizable, by predicting "how reliable (uncertainty)" the recognition results of the AI model (image recognition model).
This technology has been recognized internationally and was adopted by UAI2023 (The Conference on Uncertainty in Artificial Intelligence), a top conference on AI and machine learning technology. It will be presented at the plenary session in Pittsburgh, USA from July 31, 2023 to August 4, 2023.
Figure 1 An example of an unknown object being forcibly labeled with the wrong label.
Semantic segmentation*1, which is one of the image recognition AIs, is a technology that is indispensable in a wide range of fields such as mobility, manufacturing, and medical, as it can estimate the area of an object at the pixel level in an image.
Although it is possible to accurately estimate environments and objects similar to those at the time of learning, it is difficult to have an AI model learn all objects that exist in the real environment.
When encountering an unknown object that does not exist in the training data, forcing the AI model to apply it from among the objects it already knows may reduce the recognition performance of the AI model and lead to unexpected false positives has been a challenge so far.
For example, the objects indicated by the arrows in Figure 1 (upper left image: beer case, lower left image: dog) are unknown objects that the AI model has not learned. The part is a car (dark blue), the rest is a road (purple), and the dog is partly a tree (green) and partly a road (purple).
Especially in AI models installed in in-vehicle cameras, misrecognition of "road surface" can lead to serious accidents, and technology to prevent misrecognition of unknown objects is required.
Figure 2 Semantic segmentation model with FlowEneDet added to the latter stage
We proposed a flow-based generative model, FlowEneDet, which is a network that estimates how confident an AI model is in its recognition results (uncertainty of recognition results).
A flow-based generative model is a model that can express complex distributions as a composition of inversely transformable functions, making it possible to accurately model the distribution of training data. This modeling makes it possible to separate “objects that the AI model knows” and “unknown objects”.
Furthermore, in order to separate unknown object (OOD) and in-distribution misclassification (IDM) with higher accuracy, we proposed a combination with an energy-based model that models data density. By adding this FlowEneDet after the normal semantic segmentation model, it can be extended to a segmentation model that can estimate the uncertainty of recognition results without time-consuming retraining on the segmentation model side.
FlowEneDet itself is a model that handles low-dimensional free energy in the energy-based model, so it has a low-complexity architecture, and it can handle unknown objects (OOD) and in-distribution misclassification (IDM) without significantly increasing training and evaluation costs. simultaneously. (Figure 2)
Figure 3 Evaluation results of the proposed method. In the Ground Truth, Conventional Method, and Proposed Method, the red color represents unknown object and in-distribution misclassification, the blue color represents known object, and the other colors represent undetermined areas.
We added the newly developed FlowEneDet after the pre-trained semantic segmentation models, and performed performance evaluations benchmark datasets*2. We have achieved results that surpass conventional IDM/OOD detection methods *3. (Figure 3)
FlowEneDet can improve the performance and reliability of AI models by preventing false recognition of unknown objects that they have not learned, which AI models often encounter in real environments.
Panasonic HD will continue to accelerate the implementation of AI in society, and promote research and development of AI technology that will contribute to helping our customers live and work on site.
*1: One of major image recognition tasks. The task of recognizing an object area on a pixel-by-pixel basis and associating what object (label) is shown in each pixel. DeepLabV3+ and SegFormer are typical AI models for semantic segmentation.
*2: Cityscapes, Cityscapes-C, FishyScapes, SegmentMeIfYouCan
*3: Compared to conventional methods such as Monte Carlo Dropout (MCD) and Standardized Max Logits (SML), the false discovery rate (FPR95) at a true positive rate of 95% was reduced by 20~50%.
・“Concurrent Misclassification and Out-of-Distribution Detection for Semantic Segmentation via Energy-Based Normalizing Flow”
https://arxiv.org/abs/2305.09610
This research is the result of research by Denis Gudovskiy of Panasonic R&D Center of America, Tomoyuki Okuno and Yohei Nakata of Panasonic HD, Technology Division.
・Official PyTorch code for UAI 2023 paper "Concurrent Misclassification and Out-of-Distribution Detection for Semantic Segmentation via Energy-Based Normalizing Flow" https://github.com/gudovskiy/flowenedet
・Panasonic×AI WEBsite
https://tech-ai.panasonic.com/en/
About the Panasonic Group Founded in 1918, and today a global leader in developing innovative technologies and solutions for wide-ranging applications in the consumer electronics, housing, automotive, industry, communications, and energy sectors worldwide, the Panasonic Group switched to an operating company system on April 1, 2022 with Panasonic Holdings Corporation serving as a holding company and eight companies positioned under its umbrella. The Group reported consolidated net sales of 8,378.9 billion yen for the year ended March 31, 2023. To learn more about the Panasonic Group, please visit: https://holdings.panasonic/global/ |
The content in this website is accurate at the time of publication but may be subject to change without notice.
Please note therefore that these documents may not always contain the most up-to-date information.
Please note that German, French and Chinese versions are machine translations, so the quality and accuracy may vary.