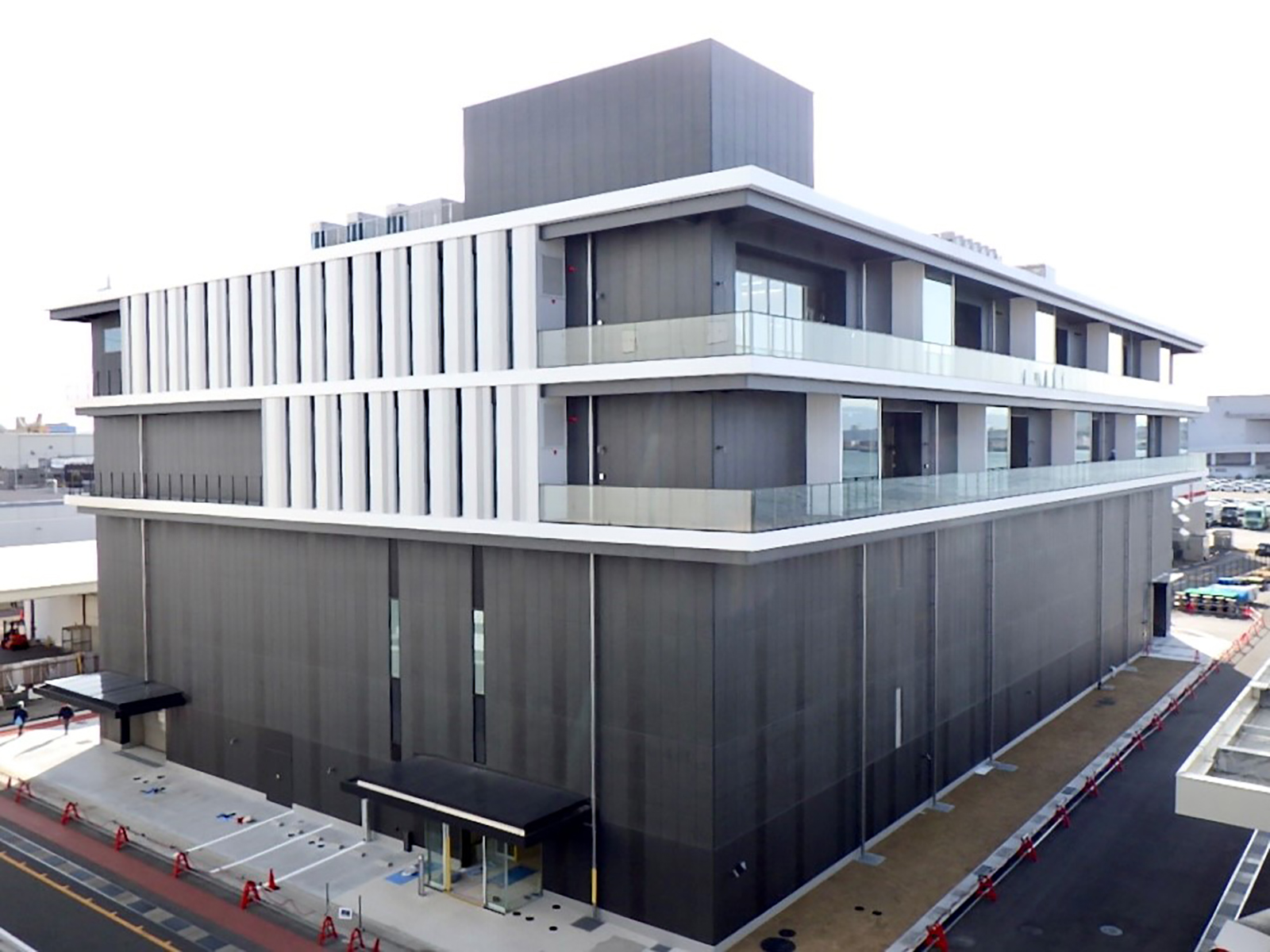
Apr 11, 2024
- Company
- Press Release
- EV Solutions
- Operating company
- Automotives & Mobility
May 23, 2023
Company / Press Release
Osaka, Japan - Panasonic Holdings Corporation (hereinafter referred to as Panasonic HD) has developed a new technology that can reduce the cost of data preparation (collecting and annotating large amount of datasets) by half while suppressing the deterioration of object detection accuracy.*1
In recent years, the implementation of AI has progressed in various fields such as public facilities and automobiles, supporting the safety and security of life and work. A large amount of training data is required to develop an AI model capable of detecting objects such as people and cars from images accurately. In each case, it is necessary to acquire a large amount of training data and annotate objects in the image each time. For this reason, a great amount of time and money is required to prepare data when deploying developed AI to various sites with different environments, and the demand for technology that can reduce data preparation costs is increasing.
The newly developed Few-shot Domain Adaptation technology*2 enables the deployment of AI models to other sites with high accuracy, using significantly less training data than previously required, even for sites with significantly varied environments. Aiming to apply this technology to a wide range of businesses and solutions for the Panasonic Group, we are proceeding with demonstration experiments using a variety of field data. We have been able to demonstrate the effectiveness of this method in object detection.
To realize an accurate AI model, it is essential to prepare a large amount of training data through data collection and annotation, which requires a lot of time and money. In order to solve this dilemma, technologies that realize high-performance AI models even with a small amount of data attract attention in the research community.
One of them is "Few-shot Domain Adaptation", which adapts the prior knowledge of an AI model trained on a large amount of publicly available labeled data (source domain data) to a different domain with only a few labeled examples (target domain). This approach is useful when labeled data in the target domain is scarce or expensive to obtain, since only a small amount of on-site data needs to be prepared. However, when the appearance of the source domain and target domain is significantly different, for example, with RGB images (source) and infrared images (target), the conventional method cannot fill the knowledge gap (domain gap)*3 between them. This leads to a deterioration in object detection accuracy.
Therefore, to bridge the domain gap created when the target domain differs significantly from the source domain, we developed a new Few-shot Domain Adaptation algorithm with a data augmentation method that synthesizes multiple images. In this method, the domain gap is reduced by replacing parts of the image of the source domain and the image of the target domain. In addition to simply replacing images, it also considers the position and existence probability of objects in the image by using the area information of the objects (cars, people, etc.) in the image to replace objects of the same type with each other (Fig. 1 (a)).
We also used adversarial learning to encourage AI models to recognize features common to both domains. Adversarial learning is a machine learning technique that trains a model by having it compete with another model that tries to deceive it. We used this to train an AI model to identify the domain of each pixel, while updating the AI model to intentionally fail to identify the domain. Eventually, the AI model could no longer distinguish between the source and target domains and was able to recognize features common to both domains (Fig. 1 (b)).
Figure 1. Configuration of the proposed method ((a) Object aware Cross-Domain CutMix (OCDC), (b) OCDC-based Discriminator Label (OCDCDL))
Through this ingenuity, we have realized a Few-shot Domain Adaptation method that is effective even when the appearance of the source domain and the target domain differ significantly, which can be difficult to address with conventional methods (Fig. 2).
Figure 2. Example of detection results in far-infrared image of conventional methods ((a), (b)), proposed method (c), and ground truth (d) when training data is reduced to 1/16 (upper) and 1/64 (lower) of the original number of images. (Quoted from paper [1])
The newly developed Few-shot Domain Adaptation method allows for the deployment of AI models to other sites with high accuracy, using much less training data than was previously required, even in environments with large domain gaps and accelerates the social implementation of AI technology that solves problems in daily life and society.
Furthermore, in use cases where it is difficult to control the acquisition conditions of training data, highly accurate AI models can be provided in a short time and at low cost, so it can be expected to contribute to, for example, shortening implementation period for on-site solutions that have various sensing targets and situations (differences in appearance, camera position, lighting conditions, etc.) for each installation site and reducing the development time of recognition technology for IR surveillance in outdoor/dark places. We will continue to work on the research and development of AI technology that contributes to the happiness of our customers.
Part of this technology was presented at the prestigious top conference ACCV*4 in the fields of image recognition and computer vision. It is a great honor to have had our research be recognized internationally in the research area essential for the social implementation of AI.
*1 As of May 23, 2023, we demonstrated using public dataset that our method can achieve almost the same performance with half the data compared to the conventional one.
*2 Machine learning technique that adapts knowledge obtained from a dataset with sufficient supervised labels (a collection of data in one environment is called a domain) to train another dataset (target domain) acquired in different environment with insufficient information in order to obtain a highly accurate recognition model even in the target domain.
*3 Differences in distribution between multiple datasets (domains) due to differences in imaging sensors, environments, brightness, etc.
*4 The Asian Conference on Computer Vision (ACCV) is an authoritative top conference in the fields of image recognition and computer vision. Known as a place for AI researchers around the world to present and discuss the latest results, in 2022 the acceptance rate is only 33%.
[1] "Few-shot Adaptive Object Detection with Cross-Domain CutMix"
(This research is the result of a collaboration between Y. Nakamura and Y. Ishii of Panasonic HD, and Professor T. Yamashita of Chubu University.)
https://openaccess.thecvf.com/content/ACCV2022/html/Nakamura_Few-shot_Adaptive_Object_Detection_with_Cross-Domain_CutMix_ACCV_2022_paper.html
Panasonic×AI Website
https://tech-ai.panasonic.com/en/
About the Panasonic Group Founded in 1918, and today a global leader in developing innovative technologies and solutions for wide-ranging applications in the consumer electronics, housing, automotive, industry, communications, and energy sectors worldwide, the Panasonic Group switched to an operating company system on April 1, 2022 with Panasonic Holdings Corporation serving as a holding company and eight companies positioned under its umbrella. The Group reported consolidated net sales of 8,378.9 billion yen for the year ended March 31, 2023. To learn more about the Panasonic Group, please visit: https://holdings.panasonic/global/ |
The content in this website is accurate at the time of publication but may be subject to change without notice.
Please note therefore that these documents may not always contain the most up-to-date information.
Please note that German, French and Chinese versions are machine translations, so the quality and accuracy may vary.